The Invisible Brain Behind Connectivity
Imagine a bustling city intersection at rush hour. Traffic lights direct vehicles, pedestrians navigate crosswalks, and emergency responders find the quickest path to the scene—all in a perfectly synchronized dance. Now, imagine if this system ran itself, adjusting in real-time to ensure everything moved smoothly. This is the role AI plays in wireless communication: an intelligent conductor orchestrating the symphony of signals that connect our world.
Artificial Intelligence (AI) has emerged as the invisible force behind modern wireless networks. It optimizes, predicts, and transforms connectivity, ensuring seamless interaction between billions of devices. From traffic management in smart cities to enabling real-time augmented reality (AR) applications, AI is rewriting the rules of wireless communication.
What is AI in Wireless Communication? A Closer Look
AI in wireless communication is often hailed as the revolutionary force transforming how networks operate, manage resources, and deliver services. But what exactly does this mean? In essence, AI in wireless refers to leveraging machine learning algorithms, neural networks, and advanced analytics to enable networks to do what they’ve never done before: think, adapt, and optimize autonomously.
Yet, behind the hype lies a more nuanced reality—one that deserves both excitement and a healthy dose of skepticism.
The Basics of AI in Wireless Communication
At its core, AI in wireless aims to solve one problem: complexity. Modern wireless networks handle an overwhelming volume of data, devices, and demands. Traditional rule-based systems struggle to keep up, leading to inefficiencies and bottlenecks. AI promises to turn these challenges into opportunities by making networks self-learning and adaptive.
Key Features of AI in Wireless
Feature | Promise | Reality Check |
---|---|---|
Real-Time Decision-Making | Process vast amounts of data instantly to optimize networks in real time. | Requires immense computational resources and precise algorithms to avoid bottlenecks. |
Predictive Analytics | Anticipate congestion, failures, and demand spikes. | Predictions are only as good as the data—biases or incomplete data can skew outcomes. |
Self-Optimization | Adapt dynamically to changing network conditions. | Works well for repetitive patterns but struggles with rare, unforeseen events. |
How AI Enhances Wireless Networks
1. Intelligent Resource Management: The Overworked Manager
AI is often described as the invisible network manager, tirelessly allocating resources like spectrum, bandwidth, and power. It’s a compelling vision: networks that intelligently borrow spectrum from one service to another based on real-time needs.
- The Upside: Dynamic Spectrum Allocation (DSA) systems are already proving their worth, helping 5G networks optimize frequency usage and reduce waste. Imagine a network "borrowing" underutilized Wi-Fi spectrum to handle a sudden spike in mobile data demand.
- The Downside: Such systems require constant monitoring and fine-tuning. The reliance on AI means that a single erroneous decision—due to a flawed algorithm or unanticipated network behavior—could cascade into widespread outages.
2. AI-Driven Beamforming: Precision with Caveats
Beamforming, a staple in modern wireless networks, focuses signals like a laser beam toward the user instead of broadcasting in all directions. AI enhances this capability by dynamically adjusting beams to avoid obstacles or interference, ensuring strong, low-latency connections.
- Why It Matters: Beamforming is critical for applications like autonomous vehicles or remote surgeries, where even a millisecond delay can have serious consequences.
- The Risk: Over-reliance on AI-driven beamforming introduces vulnerability. In dense urban environments, where signals bounce unpredictably, AI might overcompensate, leading to coverage gaps or inefficiencies.
3. Predictive Maintenance: The Network's Fortune Teller
One of AI’s most celebrated applications is its ability to foresee problems before they occur. By analyzing historical data and real-time performance metrics, AI can identify anomalies that signal impending hardware failures or network downtimes.
- Success Story: AI-driven maintenance systems in telecom have already reduced tower outages by flagging subtle performance changes that indicate a failing component.
- Challenges: These predictions depend heavily on high-quality data. In regions where data collection is inconsistent or incomplete, the effectiveness of predictive maintenance diminishes.
Balancing Potential with Reality
AI in wireless communication is not a magic wand—it’s a sophisticated tool with incredible potential and notable limitations. Its strengths lie in automating repetitive, data-heavy tasks, optimizing resource allocation, and providing predictive insights. However, its reliance on data, computational power, and human oversight cannot be ignored.
A Final Thought: The Human Factor
AI’s role in wireless networks isn’t to eliminate human involvement but to redefine it. As networks grow smarter, engineers and operators will need to focus on building better algorithms, interpreting AI-driven insights, and ensuring ethical, transparent use of these technologies.
The question isn’t whether AI will transform wireless communication—it already has. The real question is whether we’re prepared to handle the challenges that come with handing over so much control to intelligent machines.
A Brief History of AI in Wireless
1970s–1990s: Theoretical Foundations
Early efforts in applying artificial intelligence to wireless communication were focused on solving specific, repetitive tasks using rule-based systems. These foundational efforts were driven by the need to improve efficiency in network management and operations.
- Frequency Allocation: Early AI algorithms helped automate the assignment of frequency bands to different users and networks. This was crucial as radio spectrum usage began to grow with the expansion of mobile communication.
- Example: Rule-based systems in the 1980s were used in GSM (Global System for Mobile Communications) planning to ensure minimal interference and optimize frequency reuse.
- Call Routing Optimization: Early expert systems were designed to analyze network traffic patterns and reroute calls dynamically to reduce congestion. These systems could react to predefined thresholds but lacked the ability to learn and adapt.
- Example: AT&T implemented heuristic-based systems in the late 1980s to improve routing efficiency across its growing cellular network.
2000s–2010s: Machine Learning Emerges
With the advent of machine learning (ML), wireless systems began transitioning from rigid, rule-based algorithms to data-driven approaches capable of learning and adapting to dynamic conditions.
- Pattern Recognition in Traffic Management: ML models were deployed to identify patterns in network traffic, predicting peak usage times and allowing for proactive resource allocation.
- Example: In the mid-2000s, ML techniques like k-means clustering were used by telecom operators to segment network usage patterns, improving load balancing and reducing bottlenecks.
- Fault Prediction and Diagnostics: Wireless operators began using supervised learning to analyze historical network performance data, enabling predictive maintenance and minimizing downtime.
- Breakthrough: By the late 2000s, predictive algorithms could identify early warning signs of equipment failure, such as battery degradation in remote towers.
- Neural Networks and Real-Time Optimization: The 2010s saw the introduction of deep learning models, particularly neural networks, which could model complex interactions between network parameters. This enabled real-time adjustments to improve throughput and reduce latency.
- Example: In 2014, Google used neural networks to optimize traffic routing in its global data centers, a concept that later influenced telecom operators in managing network congestion.
2020s: The AI Revolution in 5G and Beyond
AI has become an indispensable element of 5G networks, transforming how networks operate, scale, and deliver services.
- Dynamic Spectrum Management: AI-driven systems now allocate spectrum resources in real time based on demand, ensuring efficient utilization and reducing interference.
- Example: In 2022, Nokia implemented AI-based Dynamic Spectrum Sharing (DSS) to allow 4G and 5G users to share spectrum seamlessly.
- Energy Efficiency Enhancements: AI models analyze energy consumption patterns across network components, dynamically switching off unused resources or redirecting traffic to more efficient nodes.
- Example: Ericsson introduced AI-powered energy-saving modes for 5G base stations in 2021, reducing operational costs by up to 15%.
- AI for Network Security: AI algorithms now detect and mitigate threats in real time, identifying anomalies that may signal cyberattacks or unauthorized access.
- Example: In 2023, AI-based anomaly detection systems helped telecom operators thwart DDoS attacks on 5G networks, ensuring service continuity during major events.
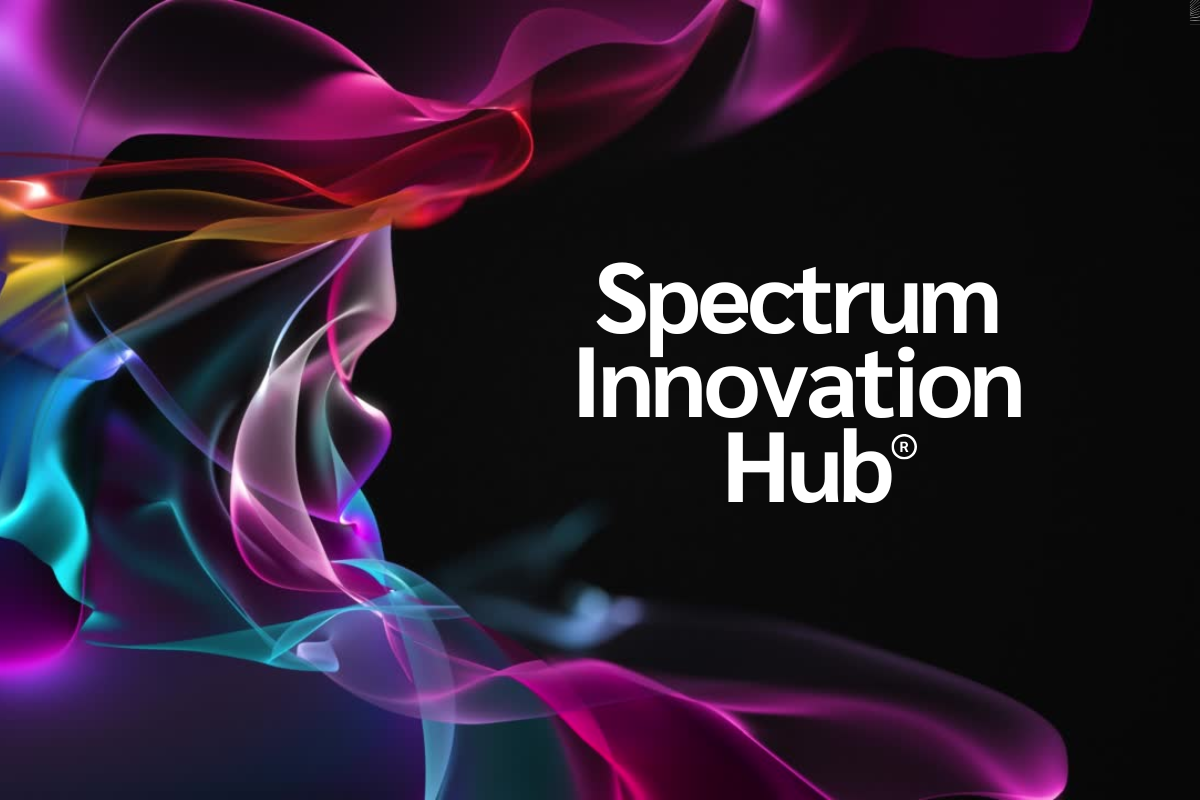
- Foundational Role in 6G: As the development of 6G networks progresses, AI is being designed into the system architecture from the ground up. Key use cases include:
- Holographic Communication: Real-time holographic calls for telemedicine and remote collaboration.
- Intelligent IoT Ecosystems: Billions of connected devices self-organizing and optimizing resource usage with minimal human intervention.
The integration of AI into wireless communication has transformed it into a proactive, self-optimizing system, paving the way for the hyper-connected world of 6G and beyond.
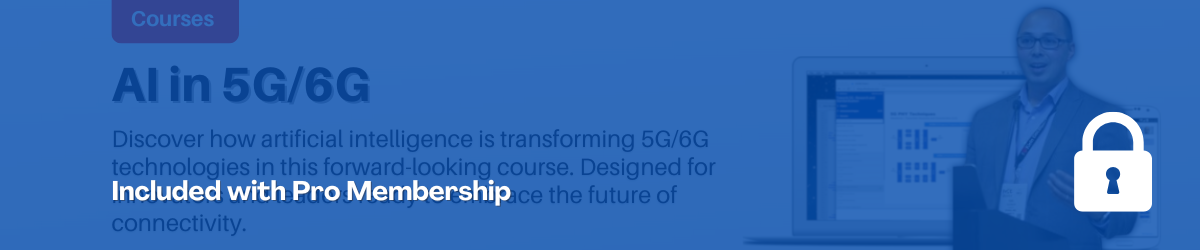
Applications of AI in Wireless Communication
1. Smart Cities
AI-powered wireless networks are the backbone of smart cities, transforming how urban infrastructure operates by improving efficiency, safety, and quality of life.
AI in Action: Smart City Comparisons
City | AI Application | Impact |
---|---|---|
Barcelona | Smart Traffic Lights | 20% reduction in traffic congestion |
Singapore | Predictive Water Management | 10% less water waste |
Dubai | AI-Powered Surveillance for Safety | 25% faster crime response times |
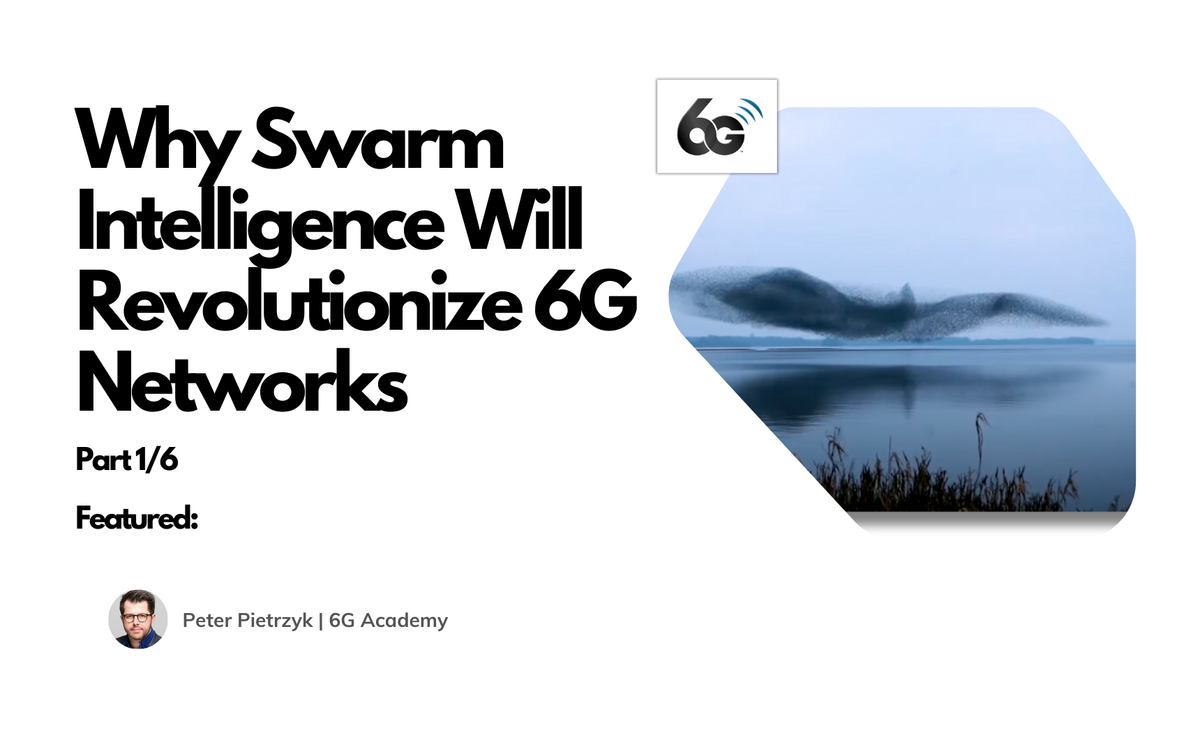
2. Industrial Automation
Factories are leveraging AI-driven wireless systems to automate production lines, improve precision, and reduce costs.
In August 2024, BMW Group Plant Spartanburg collaborated with California-based robotics company Figure to test the integration of humanoid robots, specifically the Figure 02 model, into their production line. These robots successfully performed tasks such as inserting sheet metal parts into fixtures for chassis assembly, demonstrating significant dexterity and precision. This initiative aims to alleviate workers from ergonomically challenging tasks, enhancing both efficiency and workplace safety. BMW is evaluating the potential for broader application of such robots in their manufacturing processes.
AI vs. Traditional Systems: A Quick Comparison
Feature | Traditional Systems | AI-Driven Systems |
---|---|---|
Maintenance | Reactive | Predictive |
Downtime | High | Minimal |
Resource Allocation | Fixed | Adaptive |
Cost | Higher due to inefficiency | Lower with AI-driven optimization |
3. Autonomous Vehicles
AI-enhanced 5G networks are critical for self-driving cars, enabling them to process and share data with near-zero latency.
Example: In 2023, a fleet of AI-powered delivery robots in San Francisco used 5G connectivity to navigate busy streets, avoiding accidents and delivering pizza faster than traditional couriers.
Applications of AI in Wireless Communication: AI in 3GPP Systems
AI in wireless networks isn’t just a buzzword—it’s becoming the brain behind 3GPP systems. From managing radio frequencies to ensuring seamless connectivity in dense urban environments, AI transforms static networks into dynamic, self-optimizing systems. With every 3GPP release, AI integration becomes more sophisticated, and pilot projects worldwide showcase its tangible impact.
AI in Radio Access Networks (RAN): Smarter Connectivity
Radio Access Networks (RANs) are where wireless communication begins. AI has revolutionized this domain by enabling real-time resource management and interference mitigation. Starting with 3GPP Release 17, AI-driven enhancements like the RAN Intelligent Controller (RIC) have laid the foundation for smarter networks.
AI Capability | Impact on RAN | Real-World Example |
---|---|---|
Dynamic Beamforming | AI optimizes signal paths, reducing interference. | Vodafone's AI-piloted beamforming improved signal quality in London by 20%. |
Resource Allocation | Predicts traffic patterns and pre-allocates resources. | A pilot in Japan reduced urban call drops during rush hours by 15%. |
Energy Optimization | Dynamically powers down unused components. | AI energy-saving pilots by Ericsson cut energy costs by 10%. |
These AI capabilities ensure that even during high-demand events, like concerts or sports games, RANs operate without hiccups.
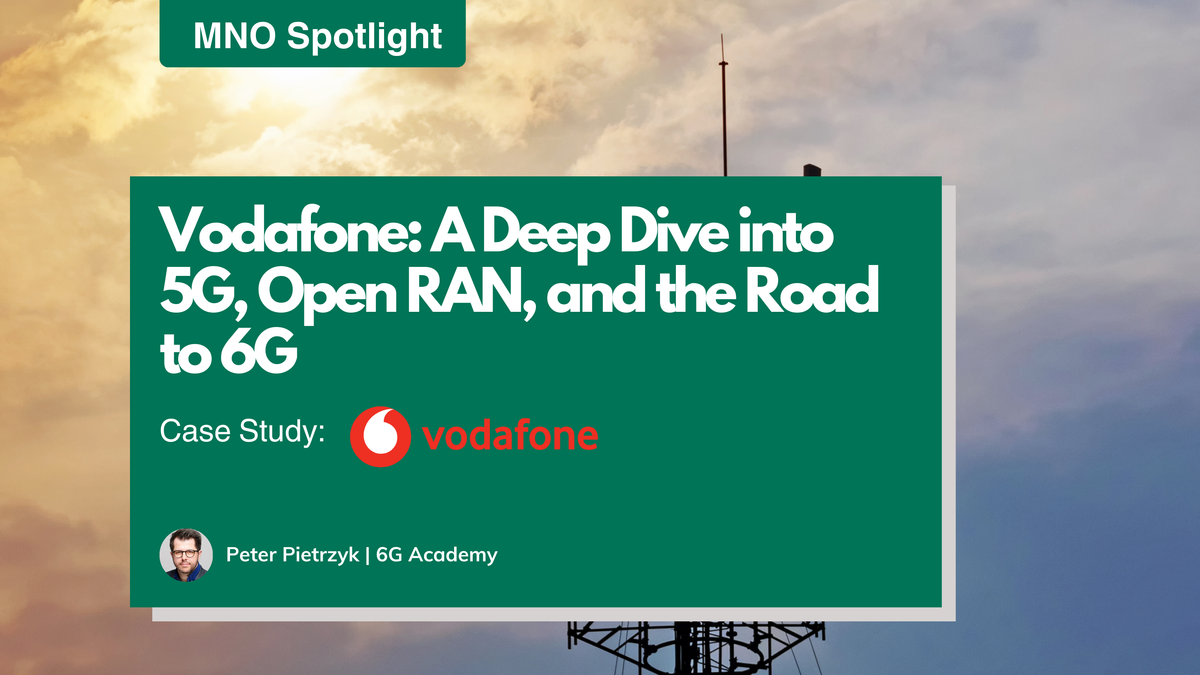
Network Slicing: One Network, Infinite Possibilities
Network slicing is one of the most exciting features of 5G, introduced in Release 15 and refined in Release 16. AI plays a pivotal role in managing these slices, tailoring them to the unique requirements of applications like IoT, AR/VR, and autonomous vehicles.
Slice Type | Key Application | AI Contribution |
---|---|---|
IoT Slices | Low-power, wide-area connections. | AI ensures energy-efficient connectivity for smart sensors. |
High-Bandwidth Slices | AR/VR and video streaming. | Dynamically adjusts bandwidth to avoid lags. |
Low-Latency Slices | Autonomous driving and robotics. | AI predicts traffic spikes for uninterrupted communication. |
AI-Enhanced Core Networks: The Backbone of Security and Scalability
The 5G Core, introduced in Release 15, uses a flexible Service-Based Architecture (SBA). AI now enhances this core by ensuring traffic flows securely and efficiently.
AI Feature | Functionality | Outcome |
---|---|---|
Anomaly Detection | Monitors traffic for unusual patterns. | Prevents DDoS attacks and service disruptions. |
Dynamic Scaling | Allocates resources based on demand. | Scales services during live-streaming events. |
Predictive Maintenance | Analyzes system performance to prevent failures. | Reduces downtime by 20% in pilot implementations. |
Energy Efficiency: Toward Greener Networks
As networks grow, so do their energy demands. AI is helping 3GPP systems align with sustainability goals by optimizing energy usage without compromising performance.
AI Feature | How It Works | Pilot Outcome |
---|---|---|
Energy-Saving Modes | AI predicts low-demand periods and powers down unused resources. | Reduced energy consumption by 15% in Ericsson’s 2022 pilot in Asia. |
Load Forecasting | AI models traffic patterns to optimize resource usage. | Avoided 10% energy waste in urban deployments. |
Renewable Energy Integration | AI aligns network usage with renewable energy availability. | Improved green energy utilization by 12%. |
These efforts not only reduce costs but also pave the way for environmentally friendly network expansion.
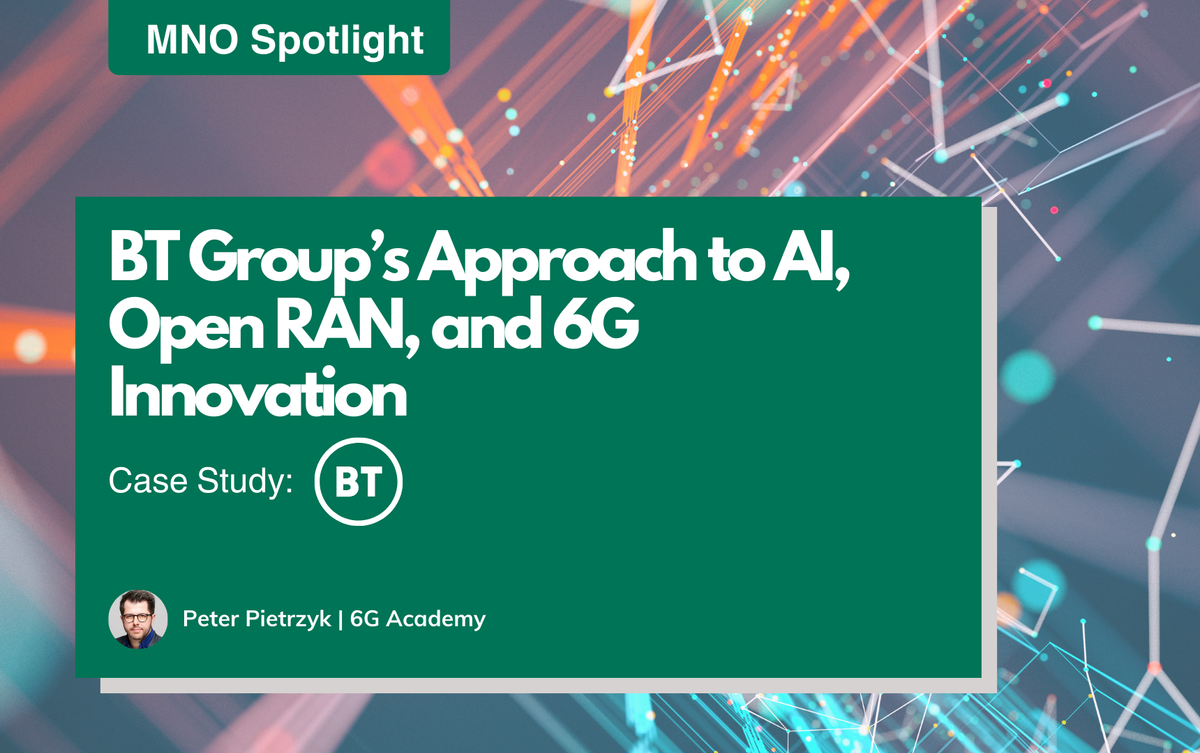
Key Takeaways
AI in 3GPP systems isn’t theoretical—it’s transforming how wireless networks operate globally. From smarter RANs to secure and scalable core networks, AI enhances every layer of connectivity. With more pilot projects and real-world implementations rolling out, it’s clear that AI is the driving force behind the future of wireless communication.
Challenges in AI-Driven Wireless Communication
AI promises to transform wireless communication, but it’s not without its hurdles. From data privacy concerns to computational bottlenecks, these challenges expose the complex interplay between innovation and practicality. Addressing them isn’t just a technical necessity—it’s a moral and ethical imperative for ensuring fair and reliable networks.
1. Data Privacy: The Cost of Intelligence
To function effectively, AI systems in wireless networks rely on massive datasets—traffic patterns, user behaviors, and environmental conditions. However, this reliance comes with significant privacy concerns.
- The Problem: Centralized AI systems often require raw data, which could expose sensitive user information to breaches or misuse. The sheer scale of data collection amplifies risks, making privacy not just a technical issue but also a regulatory and ethical one.
- Example: In 2023, privacy advocates raised concerns about telecom providers using customer data to train AI models without clear consent.
- Proposed Solution: Privacy-preserving AI techniques, such as federated learning, allow networks to train AI models on decentralized data. This means data never leaves the user’s device, minimizing exposure risks.
- Reality Check: While federated learning reduces privacy risks, it’s computationally intensive and requires significant bandwidth—ironically adding strain to the very networks it aims to improve.
The Takeaway: Privacy-preserving AI is a promising approach, but implementing it at scale while maintaining performance is an ongoing challenge. Striking a balance between innovation and user rights will require continuous refinement and transparency.
2. Computational Complexity: The Power Problem
AI models, particularly deep learning algorithms, demand immense computational resources. For wireless networks, this translates into significant energy consumption and infrastructure costs.
- The Problem: Training and running AI models centrally can create latency issues and overload network cores. This challenge grows exponentially as networks expand to support IoT devices and real-time applications like AR/VR.
- Proposed Solution: Edge AI—processing data closer to the devices rather than in centralized data centers—offers a way to distribute computational loads, reduce latency, and improve efficiency.
The Takeaway: AI’s computational appetite is a double-edged sword. While edge AI offers a path forward, the industry must consider its environmental and economic impact as it scales.
3. Bias in Algorithms: Unintended Inequities
AI is only as good as the data it learns from, and that data often reflects the biases and inequalities of the real world. In wireless networks, biased AI can lead to unfair resource allocation, such as favoring certain users or locations over others.
- The Problem: Training datasets for AI models might underrepresent specific demographics or usage scenarios, leading to skewed outcomes. For instance, rural users could face worse connectivity because urban environments dominate training data.
- Proposed Solution: Continuous monitoring, diverse training datasets, and transparency in algorithm design can mitigate bias. AI systems should also include mechanisms for detecting and correcting unintended inequities.
- Reality Check: Creating truly unbiased datasets is nearly impossible. Moreover, the effort to monitor and correct bias introduces additional layers of complexity and cost.
The Takeaway: While bias in AI is a technical challenge, it’s also a societal one. Without rigorous oversight, AI could inadvertently deepen digital divides instead of bridging them.
The Balancing Act
The challenges of AI-driven wireless communication aren’t insurmountable, but they require a careful, multi-pronged approach:
Challenge | Opportunity | Reality Check |
---|---|---|
Data Privacy | Federated learning ensures privacy. | High computational and bandwidth requirements. |
Computational Complexity | Edge AI reduces latency and central bottlenecks. | Costly infrastructure upgrades are needed. |
Bias in Algorithms | Diverse datasets and transparency improve fairness. | Eliminating bias entirely may never be feasible. |
Final Thought: Progress with Caution
AI in wireless communication is a powerful tool, but it’s not a silver bullet. As we push forward, we must confront these challenges with transparency, innovation, and humility. Only then can we unlock AI’s full potential while minimizing its risks—ensuring the networks of the future are not only smarter but also fairer and more secure.
The Future of AI in Wireless Communication
The wireless networks of today are already marvels of technology, but the networks of tomorrow promise to redefine the boundaries of connectivity. With the integration of AI into 6G and beyond, the role of wireless communication will shift from merely connecting devices to enabling intelligent, immersive, and adaptive ecosystems. However, with such transformative potential comes equally significant challenges and uncertainties.
6G and Beyond: The AI Leap
Holographic Communication: A New Dimension of Interaction
Imagine sitting across from a loved one, seeing them as a lifelike 3D hologram, even if they’re halfway across the world. AI-driven 6G networks aim to make this a reality.
- How AI Enables It: Real-time processing of massive data streams, predictive bandwidth allocation, and low-latency optimizations are all critical for delivering seamless holographic communication.
- The Challenge: Transmitting 3D holograms requires terabytes of data per second. AI must not only manage this data but also ensure it reaches users without lag or degradation—an immense technical hurdle.
AI-Driven IoT Ecosystems: A World in Sync
With billions of IoT devices expected to connect through 6G, AI will play the crucial role of orchestrating these devices in harmony. From smart homes and cities to industrial automation, AI will ensure devices communicate, learn, and optimize collaboratively.
- Why It Matters: Current networks struggle with the scale and diversity of IoT devices. AI-powered 6G will not only handle this complexity but also allow IoT ecosystems to self-organize and adapt dynamically.
- The Caveat: Ensuring security and preventing malicious exploitation in such vast, AI-managed systems will be a monumental task.
Reality Check: While the vision of synchronized IoT is compelling, the risk of creating highly vulnerable attack surfaces is real. Can AI outsmart the threats it enables?
Cognitive Networks: Networks That Think for Themselves
6G’s cognitive networks will be self-aware systems capable of learning from their environment, making decisions, and evolving autonomously. This goes beyond simple optimization—these networks will anticipate and respond to user needs before they’re even expressed.
- Potential: Cognitive networks could redefine personalization, offering bespoke services tailored to individual users in real time.
- Risk: Autonomy comes with unpredictability. How do we trust a system that evolves on its own? Furthermore, will the cost of building such networks make them viable only for industrial or high-income applications?
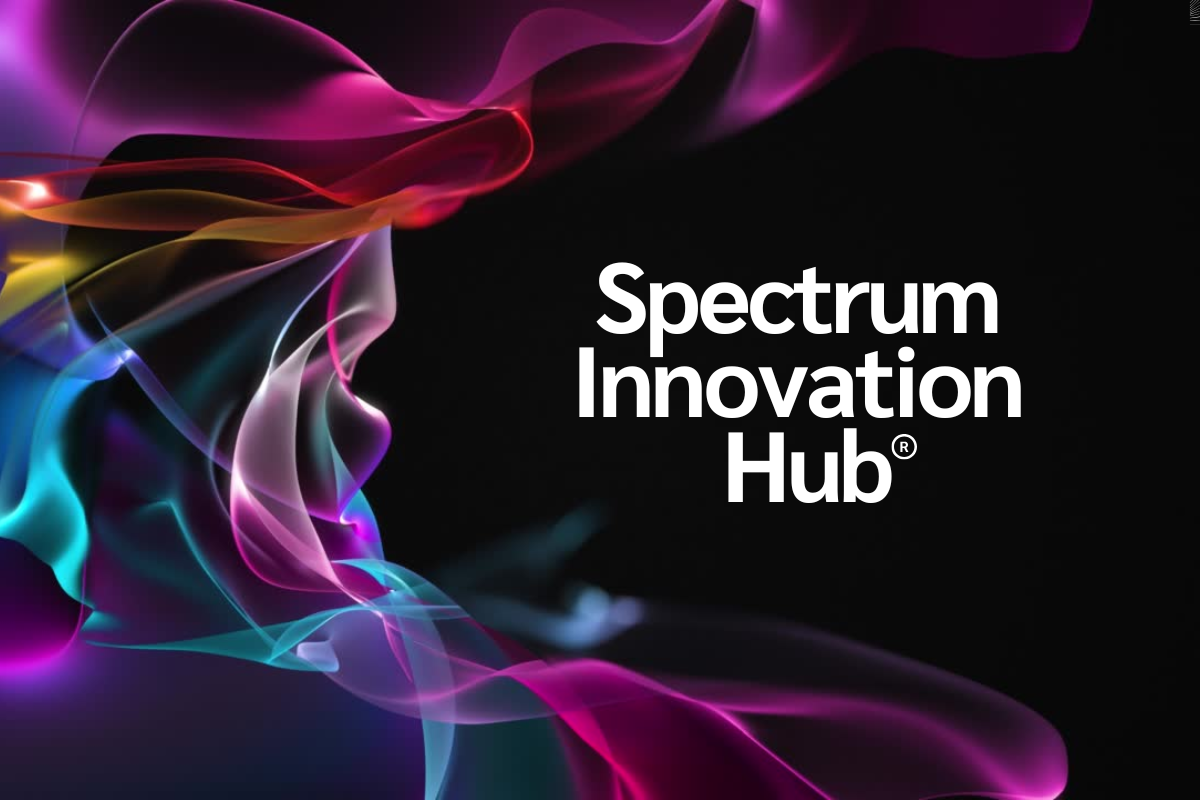
AI as the Foundation of Connectivity
AI’s role in wireless networks extends far beyond technical improvements; it’s becoming the essential framework for enabling humanity’s growing demands for data, speed, and intelligence.
Feature | Role of AI | Unresolved Questions |
---|---|---|
Resource Optimization | Ensures efficient use of spectrum and bandwidth. | How will AI handle sudden, unprecedented demand surges? |
User-Centric Design | Adapts services to individual user behavior. | How do we safeguard against invasive data use? |
Scalability | Manages billions of devices in real-time. | Can AI infrastructure scale sustainably and affordably? |
The Provocation: Is AI the Solution or the Problem?
The idea of AI as the "brain" of future connectivity is seductive, but it’s also worth asking whether we’re building a system that’s too complex to control. As networks become more autonomous, will humans lose their ability to intervene when things go wrong? Or will the very intelligence we’re building become the greatest ally in solving these challenges?
Balancing Optimism and Realism
The integration of AI into 6G and beyond promises incredible opportunities—from holographic communication to cognitive networks—but it also raises critical concerns about accessibility, security, and scalability. AI isn’t just the foundation of connectivity; it’s the lens through which we must critically assess the future of wireless communication.
The next generation of networks will be smarter, faster, and more adaptive—but whether they’ll truly serve humanity equitably depends on the decisions we make today.
Why Join 6G Academy?
The future of wireless communication is unfolding faster than ever, and staying ahead means equipping yourself with the right knowledge and skills. At 6G Academy, we’re dedicated to empowering professionals with:
- Expert-Led Courses: Dive deep into AI, 6G, and advanced wireless technologies with guidance from industry leaders.
- Cutting-Edge Insights: Stay ahead with the latest trends, breakthroughs, and practical applications shaping the next generation of connectivity.
- Career Advancement: Build your expertise, earn certifications, and position yourself as a trailblazer in the evolving wireless ecosystem.
- Exclusive Networking Opportunities: Connect with innovators, thought leaders, and peers in the global tech community.
Be part of the movement driving the next leap in connectivity. Join 6G Academy today and lead the way into the AI-powered wireless future!